Blind Source Separation and Data Fusion
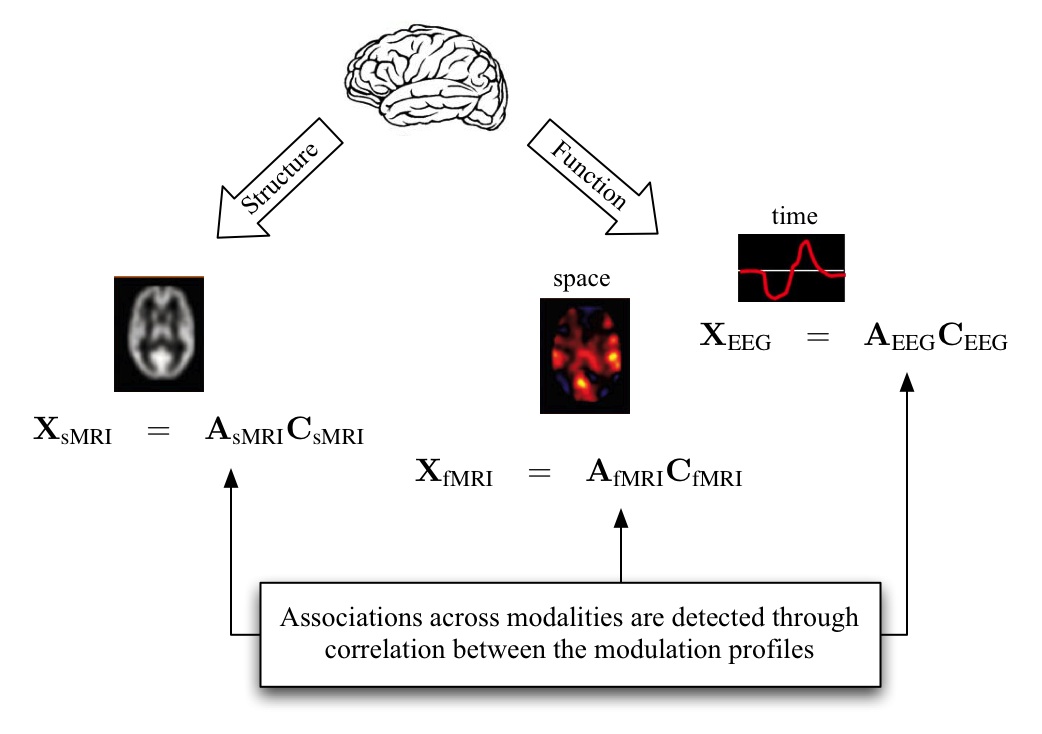
Key references:
- T. Adalı, F. Kantar, M. A. B. S. Akhonda, S. Strother, V. D. Calhoun, and E. Acar,"Reproducibility in matrix and tensor decompositions: Focus on model match, interpretability, and uniqueness," IEEE Signal Processing Magazine, vol. 39, no. 4, pp. 8-24, July 2022.
We identify critical issues for guaranteeing the reproducibility of solutions in unsupervised matrix and tensor factorizations, with an applied focus, considering the practical case where there is no ground truth. While simulation results can easily demonstrate the advantages of a given model and support a given theoretical development, when the model parameters are not known—the case in most practical problems—their estimation and performance evaluation is a difficult problem. In this article, we review the—currently rather limited—literature on the topic, discuss the proposed solutions, make suggestions based on those, and identify topics that require attention and further research.
- D. Lahat, T. Adali and C. Jutten, "Multimodal data fusion: An overview of methods, challenges, and prospects," Proc. IEEE, vol. 103, no. 9, pp. 1449-1477, Sep. 2015.
This paper provides an overview of the main challenges in multimodal data fusion across various disciplines and addresses two key issues: "why we need data fusionâ" and "how we perform it."
- T. Adali, Y. Levin-Schwartz, and V. D. Calhoun, "Multimodal data fusion using source separation: Two effective models based on ICA and IVA and their properties," Proc. IEEE, vol. 103, no. 9, pp. 1478-1493, Sep. 2015.
This paper introduces two powerful data-driven models and provides guidance on the selection of a given model and its implementation while emphasizing the general applicability of the two models.
- T. Adali Y. Levin-Schwartz, and V. D. Calhoun, "Multimodal data fusion using source separation: Application to medical imaging," Proc. IEEE, vol. 103, no. 9, pp. 1494-1506, Sep. 2015.
This paper demonstrates the application of the two models introduced in the previous paper to fusion of medical imaging data from three modalities: functional magnetic resonance imaging (MRI), structural MRI, and electroencephalography data and discusses the tradeoffs in various modeling and parameter choices.
-
T. Adali, M. Anderson, and G.-S. Fu, "Diversity in independent component and vector analyses: Identifiability, algorithms, and applications in medical imaging," IEEE Signal Processing Magazine, vol. 31, no. 3, pp. 18-33, May 2014.
In this overview article, we first present ICA, and then its generalization to multiple data sets, IVA, both using mutual information rate, present conditions for the identifiability of the given linear mixing model and derive the performance bounds. We address how various methods fall under this umbrella and give examples of performance for a few sample algorithms compared with the performance bound. We then discuss the importance of approaching the performance bound depending on the goal, and use medical image analysis as the motivating example.
Active projects:
-
Collaborative Research:CISE-ANR:CIF:Small:Learning from Large Datasets - Application to Multi-Subject fMRI Analysis
Funded by NSF CCF, Division of Computing and Communication Foundations (Grant Number: NSF-CCF 2316420)
An many disciplines today, there is an increasing availability of multiple and complementary data associated with a given problem, and the main challenge is extracting and effectively summarizing the relevant information from these large number of datasets. Joint decomposition of these datasets, arranged as matrices or tensors, provides an attractive solution to data fusion by letting them fully interact and inform each other and yields factor matrices that are directly interpretable, where the resulting factors (components) are directly associated with quantities of interest. This research will provide a powerful solution for inference from large-scale data by effectively summarizing the heterogeneity in large datasets through the definition of homogeneous subspaces such that components within a subspace are highly dependent. The methods will leverage both statistically motivated methods like the independent vector analysis and coupled tensor decompositions, and the success of the methods will be demonstrated through identification of homogeneous subgroups of subjects from neuroimaging data, thus enabling personalized medicine whose goal is to tailor intervention strategies for a given individual.
Dynamic imaging-genomic models for characterizing and predicting psychosis and mood disorders
Funded by NIH-NIMH (Grant Number: R01 MH 118695)
Disorders of mood and psychosis such as schizophrenia, bipolar disorder, and unipolar depression are incredibly complex, influenced by both genetic and environmental factors, and the clinical characterizations are primarily based on symptoms rather than biological information. Current diagnostic approaches are based on symptoms, which overlap extensively in some cases, and there is growing consensus that we should approach mental illness as a continuum, rather than as a categorical entity. Both imaging and genomic data are high dimensional and include complex relationships that are poorly understood. To characterize the available information, we are in need of approaches that can deal with high-dimensional data exhibiting interactions at multiple levels through data fusion while providing interpretable solutions (i.e., a focus on brain and genomic networks). Our approach leverages the interpretability of source separation approaches and can include additional flexibility by allowing for a combination of shallow and deep subspaces, thus leveraging the power of deep learning. We will apply the developed models to a large (N>60,000) dataset of individuals along the mood and psychosis spectrum to evaluate the important question of disease categorization. The combination of advanced algorithmic approach plus the large N data promises to advance our understanding of the nosology of mood and psychosis disorders in addition to providing new tools that can be widely applied to other studies of complex disease.
Recent related projects:
-
CIF: Small: Source Separation with an Adaptive Structure for Multi-Modal Data Fusion
Funded by NSF CCF, Division of Computing and Communication Foundations (Grant Number: NSF-CCF 1618551)
Today, there is significant activity in data fusion in disciplines spanning medical imaging, remote sensing, speech processing, behavioral sciences, and metabolomics, to name a few. A common challenge across multiple disciplines is determining how and to what degree different datasets are related, i.e., identifying the common and distinct subspaces across multiple datasets in terms of the information they provide for the given task. The current work provides a powerful solution to this key challenge enabling identification of the relationship among multiple datasets in a completely data-driven manner such that both the common and distinct subspaces within each dataset can be robustly identified and analyzed.
CRCNS: Informed Data-Driven Fusion of Behavior, Brain Function, and Genes
Funded by NIH-NIBIB (Grant Number: R01 EB 005846)
As imaging scanners improve and are able to collect images faster, more studies collecting multiple types of imaging information from the same participants are being used to study connectivity in healthy controls and pathological states. However, each existing modality for imaging the living brain can only report upon a limited domain. The goal of the second project CRCNS: Collaborative Research: Spatiotemporal Fusion of fMRI, EEG, and Genetic Data is to examine associations among fMRI, EEG, and genetic variations related to healthy and abnormal brain function. As part of this project, we develop a set of tools based on ICA that can effectively fuse the information provided by multiple imaging modalities to span a vast range of spatial and temporal scales.
CIF: Small: Collaborative Research: Entropy Rate for Source Separation and Model Selection: Applications in fMRI and EEG Analysis
Funded by NSF-CCF: Award no: 1117056 and Award no: 1116944
The focus of this research is the development of a class of powerful methods for source separation and model selection using entropy rate so that one can take both the higher-order-statistical information and sample correlation into account to achieve significant performance gains in more challenging problems. The main application domain is one that can truly take advantage of this fully combined approach: the analysis of functional magnetic resonance (fMRI) data and the rejection of gradient and pulse artifacts in electroencephalography (EEG) in concurrent EEG-fMRI data.
Collaborative Research: III: Small: Collaborative Research: Canonical Dependence Analysis for Multi-modal Data Fusion and Source Separation
Funded by NSF-IIS: Award no: 1017718 and Award no: 1016619
In this grant, the main aim is twofold. First, a number of powerful methods are developed for multi-subject (multi-set) data analysis and multi-modal data fusion based on canonical dependence analysis by significantly extending the power and flexibility of MCCA. Then, the successful application of the methods are demonstrated on a unique problem that demands these properties, namely the study of brain function and functional associations during simulated driving, a naturalistic task where data-driven methods have proven very useful.
Collaborative Research: SEI: Independent Component Analysis of Complex-Valued Brain Imaging Data
Funded by NSF-IIS (Award no: 0612076)
We develop a class of complex ICA algorithms, in particular for analysis of biomedical imaging data and demonstrate the power of joint data analysis as well as performing the analysis on the complete set of data, i.e., by utilizing both the magnitude and the phase information. We focus upon three image types, functional magnetic resonance imaging (fMRI), structural MRI (sMRI) and diffusion tensor imaging (DTI). These three imaging data provide complementary information about brain connectivity, and all can benefit from the incorporation of a complex-valued data processing approach.
Other selected references:
-
M. Anderson, G.-S. Fu, R. Phlypo, and T. Adali, "Independent Vector Analysis: Identification Conditions and Performance Bounds," IEEE Trans. Signal Processing, to appear.
- M. Anderson, X.-L. Li, and T. Adali, "Joint blind source separation with multivariate Gaussian model: Algorithms and performance analysis," IEEE Trans. Signal Processing, vol. 60, no. 4, pp. 2049--2055, April 2012.
Project team:
-
Current: Dr. Trung Vu, Ben Gabrielson, Isabell Lehmann, Clement Cosserat, and
Dr. Tulay Adali
Resources:
- MLSP-Lab Resources: Codes for the algorithms we have published.
- Fusion ICA Toolbox (FIT):
FIT is a MATLAB toolbox which implements the joint ICA and parallel ICA methods. It is used to examine the shared information between the features (SPM contrast image, EEG signal or SNP data).