Study of Brain Function
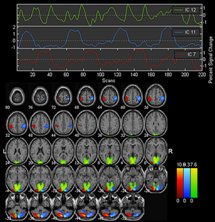
Our work is in collaboration with
Key references:
-
V. D. Calhoun and T. Adalı,"Time-varying brain connectivity in fMRI data: Whole-brain data-driven approaches for capturing and characterizing dynamic states," IEEE Signal Processing Magazine, vol. 33, no. 3, pp. 52-66, May 2016.
O The study of whole-brain functional brain connectivity with functional magnetic resonance imaging (fMRI) has been based largely on the assumption that a given condition (e.g., at rest or during a task) can be evaluated by averaging over the entire experiment. In actuality, the data are much more dynamic, showing evidence of time-varying connectivity patterns, even within the same experimental condition. In this article, we review a family of blind-source separation (BSS) approaches that have proven useful for studying time-varying patterns of connectivity across the whole brain.
- T. Adali Y. Levin-Schwartz, and V. D. Calhoun, "Multimodal data fusion using source separation: Application to medical imaging," Proc. IEEE, vol. 103, no. 9, pp. 1494-1506, Sep. 2015.
This paper demonstrates the application of the two models introduced in the previous paper to fusion of medical imaging data from three modalities: functional magnetic resonance imaging (MRI), structural MRI, and electroencephalography data and discusses the tradeoffs in various modeling and parameter choices.
-
V. D. Calhoun, R. Miller, G. Pearlson, and T. Adali, "The chronnectome: time-varying connectivity networks as the next frontier in fMRI data discovery,"
Neuron, vol. 84, no. 2, Oct. 22, 2014.
In this Perspective, we use the term "chronnectome" to describe metrics that allow a dynamic view of coupling. In the chronnectome, coupling refers to possibly time-varying levels of correlated or mutually informed activity between brain regions whose spatial properties may also be temporally evolving. We provide an overview of multivariate methods and discuss the potential these approaches offer to improve characterization and understanding of brain function.
- V. D. Calhoun and T. Adali,"Multi-subject independent component analysis of fMRI: A decade of intrinsic networks, Default Mode, and Neurodiagnostic Discovery," IEEE Reviews in Biomedical Engineering, invited contribution, vol. 5, pp. 60-73, 2012. In this overview article, we present an overview of the application of ICA to fMRI data analysis and discuss current directions and challenges.
- V. D. Calhoun and T. Adali, "Feature-based fusion of medical imaging data," IEEE Trans. Info. Tech. and Biomedicine, vol. 13, no. 5, pp. 711-720, Sep. 2009. We present an overview of feature-based approaches for fusion of medical data with emphasis on an approach that uses independent component analysis for the task.
- Functional MRI: Our Window onto the Brain, Theme Issue, IEEE Engineering in Medicine and Biology Magazine, T. Adali, and V. D. Calhoun (editors), vol. 25, no. 2, March/April 2006. This theme issue of the EMB magazine we have edited presents an overview of functional MRI data and the techniques for its analysis.
Active projects:
-
Funded by the NIH/NIMH (Grant Number: R01-MH118695)
Disorders of mood and psychosis such as schizophrenia, bipolar disorder, and unipolar depression are incredibly complex, influenced by both genetic and environmental factors, and the clinical characterizations are primarily based on symptoms rather than biological information. Our approach leverages the interpretability of source separation approaches and can include additional flexibility by allowing for a combination of shallow and deep subspaces, thus leveraging the power of deep learning.
Data-driven solutions for temporal, spatial, and spatiotemporal dynamic functional connectivity
Funded by the NIH/NIMH (Grant Number: R01-MH123610)
Existing approaches to estimate and characterize whole brain time-varying connectivity from fMRI data have shown considerable promise, with exponential growth in research in this field. We and others have developed a powerful set of tools that are now in wide use in the community. However, the impact of mental illness on brain connectivity is complex, and as we show, limitations in existing methods often result in missing important features associated with brain disorders (e.g. transient fractionation of the spatial structure of brain networks). In this project, we will address main current challenges and will develop a novel family of models that builds on the well-structured framework of joint blind source separation to capture a more complete characterization of (potentially nonlinear) spatio-temporal dynamics while providing a way to relax other limiting assumptions.
Recent related projects:
-
Collaborative Research: NCS-FO: Flexible Large-Scale Brain Imaging Analysis: Diversity, Individuality, and Scalability
Funded by the NSF (Grant Number: 1631838)
This project is designed to develop flexible data-driven multi-subject analysis methods for brain imaging data, provide new educational and outreach activities to help promote the workforce, and create a software tool to foster big data analysis of the human brain.
CRCNS: Informed Data-Driven Fusion of Behavior, Brain Function, and Genes
Funded by NIH-NIBIB (Grant Number: R01 EB 005846)
As imaging scanners improve and are able to collect images faster, more studies collecting multiple types of imaging information from the same participants are being used to study connectivity in healthy controls and pathological states. However, each existing modality for imaging the living brain can only report upon a limited domain. The goal of the second project CRCNS: Collaborative Research: Spatiotemporal Fusion of fMRI, EEG, and Genetic Data is to examine associations among fMRI, EEG, and genetic variations related to healthy and abnormal brain function. As part of this project, we develop a set of tools based on ICA that can effectively fuse the information provided by multiple imaging modalities to span a vast range of spatial and temporal scales.
Unified Multivariate Data-Driven Solutions for Static and Dynamic Brain Connectivity
Funded by NIH-NIBIB (Grant Number: R01 EB 020407)
There has been great progress in the use of functional connectivity measures to study the healthy and dis- eased brain. The fMRI community has now realized that assessment of functional connectivity has been limited by an implicit assumption of spatial and temporal stationarity throughout the measurement period. Dynamics are potentially even more prominent in the resting-state, during which mental activity is unconstrained. Using a powerful framework that builds on the well-structured framework of joint blind source separation, we make use of all available prior and statistical information---higher-order-statistics, sparsity, smoothness, sample and dataset dependence to derive a class of novel and effective dynamic models for full characterization of static and dynamic brain connectivity.
A Unified Framework for Flexible Brain Image Analysis
Funded by NIH-NIBIB (Grant Number: R01 EB 000840)
Independent component analysis has found a fruitful application in the analysis of brain imaging data, in particular for functional magnetic resonance imaging (fMRI) and electroencephalography (EEG) data. This project focuses on the development of data-driven methods for analysis of fMRI data by incorporating prior information into the estimation.
Collaborative Research: SEI: Independent Component Analysis of Complex-Valued Brain Imaging Data
Funded by NSF-IIS (Award no: 0612076)
We develop a class of complex ICA algorithms, in particular for analysis of biomedical imaging data and demonstrate the power of joint data analysis as well as performing the analysis on the complete set of data, i.e., by utilizing both the magnitude and the phase information. We focus upon three image types, functional magnetic resonance imaging (fMRI), structural MRI (sMRI) and diffusion tensor imaging (DTI). These three imaging data provide complementary information about brain connectivity, and all can benefit from the incorporation of a complex-valued data processing approach.
Selected other publications:
- Y.-O. Li, T. Adali, and V. D. Calhoun, "Estimating the number of independent components for fMRI data," Human Brain Mapping, vol. 28, pp. 1251-66, 2007. This work proposes an i.i.d sampling scheme based on an entropy rate measure to correct sample dependence effect on order selection by information theoretical criteria. The method is applied to the true fMRI data and the results are validated by studying the stability of the subsequent independent component analysis on the fMRI data. The order selection scheme is implemented in the Group ICA of fMRI Toolbox (GIFT).
- N. M. Correa, T. Adali, and V. D. Calhoun,
"Canonical correlation analysis for data fusion and group analysis: Examining applications of medical imaging data,"
IEEE Signal Processing Magazine, vol. 27, no. 4, pp. 39-50, July 2010.
The article presents an overview of the application of second-order
analysis method, CCA, to data fusion and joint data analysis.
Project team:
-
Current: Dr. Trung Vu, Hanlu Yang, Chunying Jia, Isabell Lehmann, and Dr. Tülay Adali
Resources:
-
Group ICA Toolbox (Includes GIFT and EEGIFT):
GIFT is a Matlab toolbox which implements multiple algorithms for independent component analysis and blind source separation of group (and single subject) functional magnetic resonance imaging data and electro encephalogram data.
- Fusion ICA Toolbox (FIT):
FIT is a MATLAB toolbox which implements the joint ICA and parallel ICA methods. It is used to examine the shared information between the features (SPM contrast image, EEG signal or SNP data).
- Simulated fMRI dataset: We generate a simulated fMRI-like set of components and mix them with a set of time courses to obtain a simulated fMRI-like dataset.